Superconducting Materials and Systems Center
The Superconducting Quantum Materials and Systems Center (SQMS), brings together partners including Rigetti Computing, Ames Laboratory and NASA Ames Research Center and University of Arizona with the goal of building and deploying a quantum computer at Fermilab and develop new quantum sensors based on superconducting technology. SQMS research focuses on optimizing the coherence time of a qubit, therefore increasing length of time that a quantum computer can effectively process information. Error Correction Lab contributes to the Quantum Algorithms and Theory trust of the SQMS though development of quantum error correction.
NSF CIF: Medium: Iterative Quantum LDPC Decoders
We are developing novel quantum fault tolerant decoding algorithms for finite length quantum LDPC decoders beyond belief propagation (BP), leveraging knowledge of trapping sets to surpass BP, not only in decoding error performance but also in complexity. We investigate iterative decoders for quantum codes realized solely by quantum circuits and developing machine learning algorithms for learning such quantum decoders. Theoretical techniques to analyze the decoders to derive explicit conditions on the graph that guarantees performance in terms of error correction capability are also pursued.
NSF ECCS/CCSS- 2027844: Neural Network Nonlinear Iterative LDPC Decoders with Guaranteed Error Performance and Fast Convergence
The proposed research addresses the hardware complexity, error floor and convergence problems of decoders of low-density parity check (LDPC) codes in a unified way by the following novel approaches: nonlinear message update functions, guaranteed error correction capability, neural network decoders, built-in stochastic gradient descent.
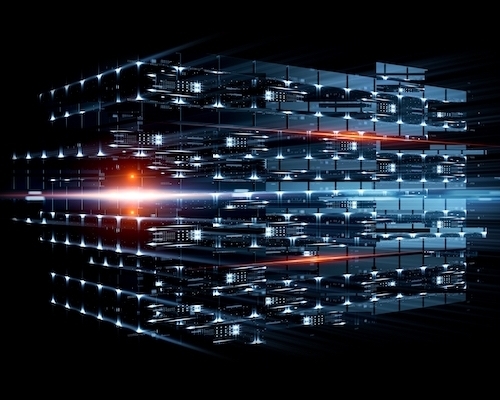
NSF-ERC – 1918635 Engineering Research Center for Quantum Networks
The Center for Quantum Networks (CQN) brings together partners is developing the first quantum network enabling fully error-corrected quantum connectivity at 10 M qubits/s over 100-km. This network will support a certified quantum fidelity of 99+%, simultaneously for multiple user pairs, supported on a backbone network built from quantum repeaters and quantum switches. The goal of the project is to quntumly connect the MIT and University of Arizona campuses in such a way that information always stays in quantum domain.
NSF ECCS-1500170: Enabling High-Speed Communication over Turbulent Free-Space Optical Links by Employing Spectral-Spatial Schemes
The goal of this project is to investigate free-space optical communication schemes by utilizing both frequency and space properties through their parallel employment within a single propagating light beam. Although this approach is highly promising, propagation through the turbulent atmosphere can drastically degrade the signal quality and thus reduce the information capacity. Therefore, an innovative approach is needed to advance solutions that can increase the information capacity and compensate for these turbulence effects.
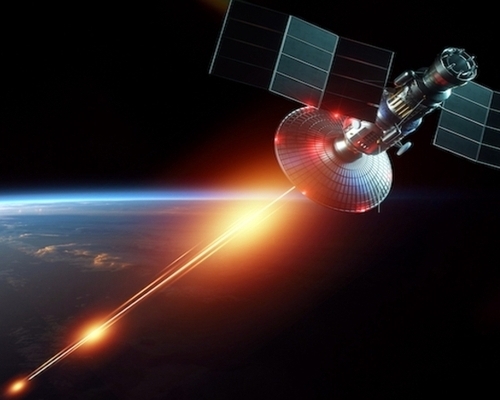
NSF-CIF-2100013: Small: Learning To Correct Errors
The beauty of codes and elegance of their decoding algorithms reflect the rich structure of the underlying mathematical objects, but also human creativity and ingenuity. In this project we investigate if, how, and how efficiently this structure and elegance can be learned by a machine, and how a discovery of new decoding algorithms and the improvement of the existing ones can be automatized. As the structure of Deep Neural Networks (DNN) in various applications, naturally supports iterative decoders of linear block codes – an important and large class of codes and decoders, – the main goal can be formulated as learning new DNN based iterative decoders that optimize: decoding probability of error and convergence speed under the decoding complexity constraints.
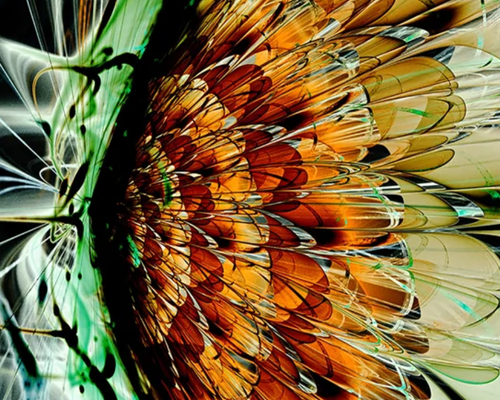
NSF-ECCS/CCSS -2052751: Collaborative Research: Secure and Efficient Post-quantum Cryptography: from Coding Theory to Hardware Architecture
The fast development of quantum processors brings the imminent need of new cryptography schemes that are secure against quantum computing attacks. In this project, we study the code-based McEliece/Niederreiter cryptosystems, especially those based on low-density and medium-density parity check (LDPC and MDPC) error-correcting codes, one of the most promising post-quantum cryptography schemes. This proposal spans cryptography, error correcting coding theory, and hardware architecture design for the code-based McEliece/Niederreiter cryptosystems. In this project we are developing efficient and all-around secure designs resisting attacks from all perspectives, including information theoretical analysis, side channel, and hardware counterfeiting.
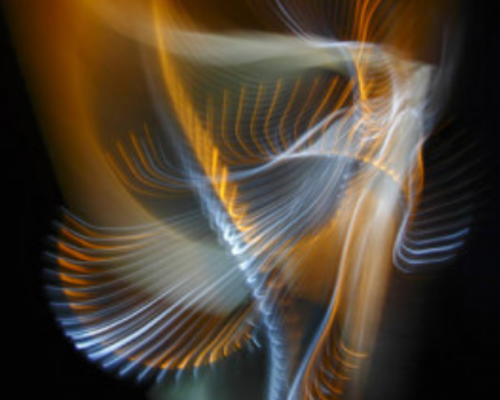
NSF CIF-2106189: Collaborative Research: CIF: Medium: QODED: Quantum codes Optimized for the Dynamics between Encoded Computation and Decoding using Classical Coding Techniques
The theory of stabilizer and subsystem quantum error correcting codes (QECCs) has laid a strong foundation for synthesizing effective QEC techniques, which correct errors as well as allow fault-tolerant logical operations on the encoded information. The core problem underlying QEC for quantum computing is the dynamics, or interplay, between decoding and logical computation. This is a challenging problem as, in general, the structure required for good and efficient decoding is not necessarily the same as that required for fault-tolerant logical operations. In this project we are developing a systematic framework for studying the mutual interaction between these primary objectives of quantum computing.
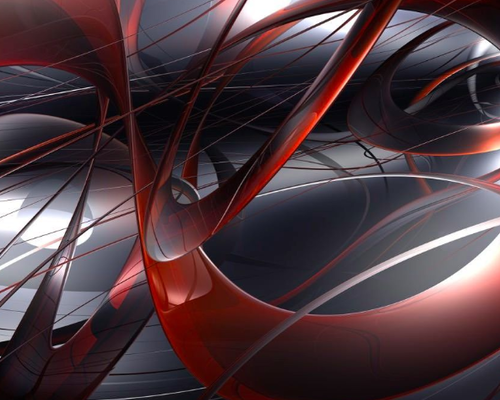
NASA: Robust Neural Network Decoders for Quantum Error Correction Systems
In this Strategic University Research Partnership Project together with colleagues at NASA Jet Propulsion Laboratory (JPL) we are developing high-performance, low-complexity, fault-tolerant decoding algorithms for quantum communications systems for enhancing JPL’s capabilities to support future communications systems. We use deep neural networks (DNNs) to learn complex codeword decision region boundaries to approach the maximum likelihood performance, while maintaining sparse weight matrices. The main challenge is that typical coding applications require extremely low error rates (10-9 or lower), many orders of magnitude lower than misclassification rates in traditional DNN applications. To keep the decoding complexity manageable for a space link, the neuron is quantized to only a few bits. In the proposed research, we are incorporate prior coding theory knowledge to improve deep learning for decoder design.
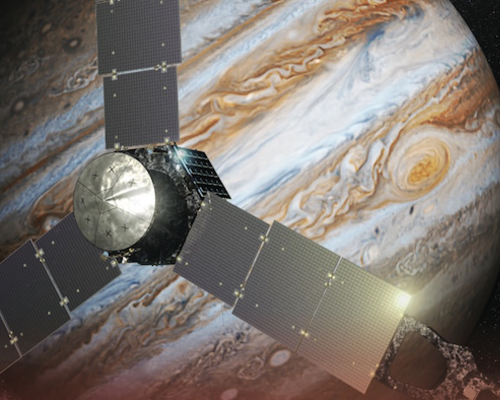
Quantum Error Correction – Synergy between Quantum Physicists and Coding Theorists
The long-term vision of this activity is to establish a platform for active discussions on problems in quantum error correction. The goal of the workshops, seminars, and special sessions that we organize is to provide foundational knowledge in quantum error correction for fault-tolerant quantum computing. We aim to complement this with talks by invited speakers working in this area, foster discussions on key open problems—both foundational and practical ones—and discuss the latest results in the field.
Seminars & Workshops:
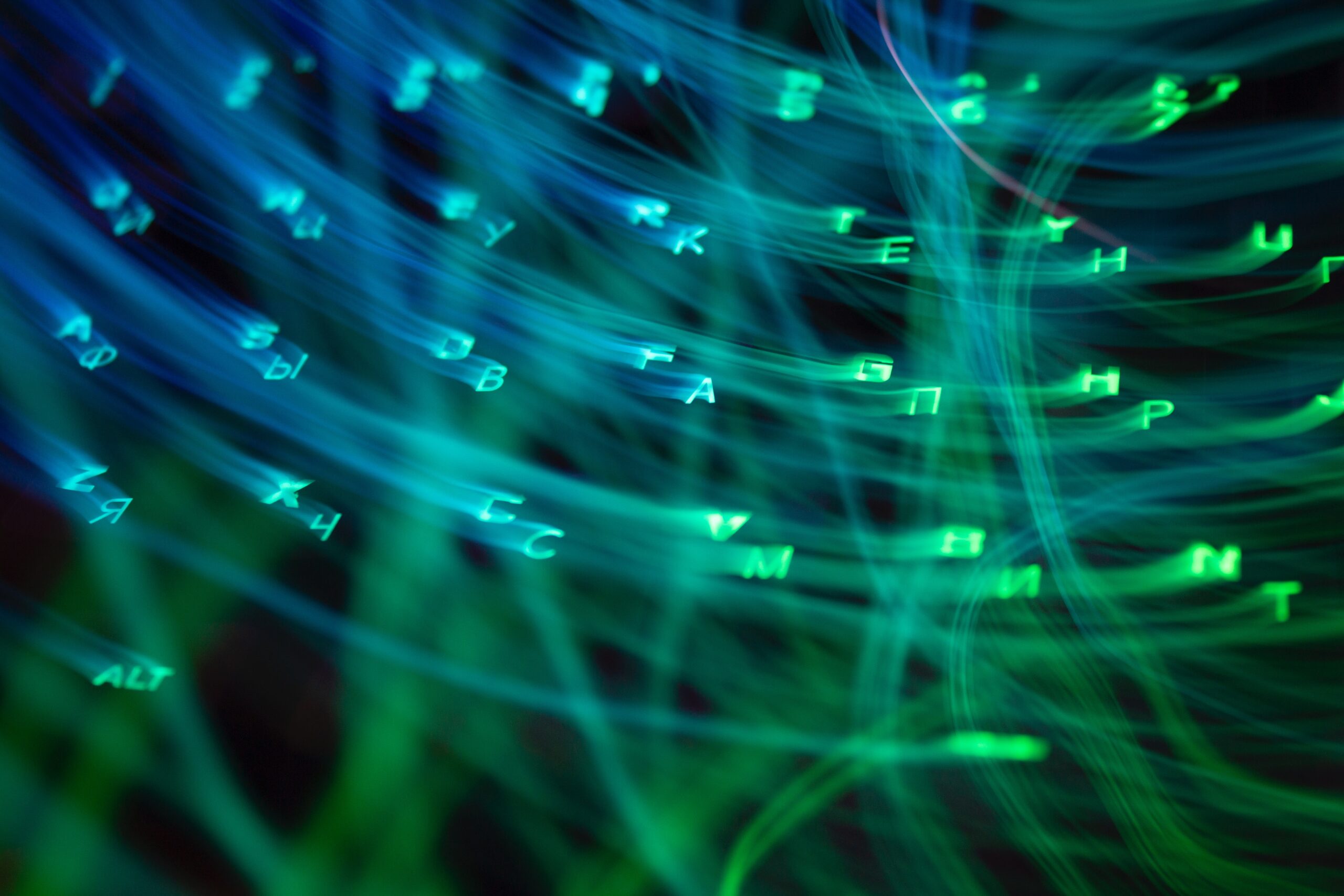